Summary
What Artificial Intelligence reveals about share price reactions?
- Using Artificial Intelligence (AI), Amundi – partnering with Causality Link and Toulouse School of Economics – is building aggregate news signals to capture the tone and popularity of company news.
- These news signals can predict share price movements in the near term for different sized companies and news types.
- This may be a promising area of development in asset management: Investors can use these news signals to build strategies that aim to achieve excess gross returns.
With the advances in natural language processing algorithms, it is now possible to extract relevant content from a large volume of textual data available in financial markets.
Using a proprietary algorithm, the Amundi Institute in partnership with the AI platform Causality Link1 and the Toulouse School of Economics, extracts content from the textual data available across the financial markets2. By searching articles, call transcripts and broker research, the AI platform identifies a company’s name, its Key Performance Indicators (KPIs), any directional change in these KPIs and the tense (past, present or future) of news statements. Using this data, an aggregate news signal is built to capture not only the positive or negative tones in news flow, but also how popular this is on any given day. The strength of the signal’s informational content is measured, as well as how and when new fundamental information is factored into share prices. Going a step further than other studies, the AI platform taps into numerous data sources and examines share price reactions across different sized companies and news types.
The findings highlight that companies’ share prices react more strongly to news about their future than to revelations about their current or past achievements. Furthermore, financial news is a greater driver of share prices than news linked to environmental, social and governance (ESG) issues, while information about smaller companies can be a more significant share price driver than news flow concerning bigger businesses. Overall, the findings bring to light the strong informational content of the news flow analysed by the Causality Link platform. In practice, given all news types are not immediately reflected in share prices, investors can use Amundi’s news signals to support strategies that aim to achieve gross excess returns.
What research has taken place?
Advances in natural language processing (NLP) algorithms are allowing investors to examine share price reactions to company news flow. Thanks to a partnership with the AI platform, Causality Link, and the Toulouse School of Economics, Amundi can analyse companies’ fundamental information, which is derived from textual data, on a large scale. News stories, call transcripts, earnings announcements and broker research are the rich sources of textual data used by Amundi’s research team. Using the Causality Link platform3, data is extracted from companies’ news statements: a company’s name, its Key Performance Indicators (KPIs), any directional changes in these KPIs and the tense (past, present or future) of news statements (see Box 1).
Box 1. An example of how content is sourced from a news statement
- Example statement: “Despite the slowdown, both Google and Windows devices saw K-12 unit growth during the fourth quarter, while Apple’s iPad volumes declined year-over-year.” (USA TODAY, at 11:30:00 PM March 25, 2018).
- After identifying Apple as the company, the algorithm detected: a fall in Apple’s transaction volume (KPI); the negative tone of the news for shareholders; and the KPI performance in relation to the fourth quarter of the previous year.
- In addition, the algorithm observed that a second company, Google, was mentioned in the statement and that its KPI unit growth also slowed during the same period.
Using this data, an aggregate news signal can be built to capture the positive or negative tone of a company’s news on any given day (e.g. a report outlining a sales volume decline (a KPI) would have a negative tone), as well as how widely the news has been distributed in the marketplace4 (see Figure 1).
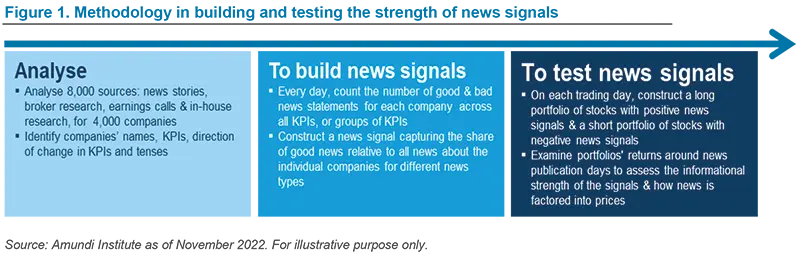
Amundi Institute’s research assesses the informational content of the aggregate news signals. We examine the returns of two portfolios on publication day, one consisting of stocks with the most positive news signals while the other is made up of a short portfolio of stocks with the most negative news signals. The same process can be applied to subsets of the data (i.e. high/low news coverage, fresh/stale news, types of news (financial/ESG), the tense of these news statements, news horizons (short term/long term) and company size (large cap/small cap).
Shinning a lens on stock price reactions
Artificial intelligence and innovative tools may help to develop new fields of research which can aid investors in extracting alpha from company news.
Amundi’s study shows that aggregate news signals are informative about company-specific fundamental news and that a long-short strategy can produce positive excess returns up to the day after the news publication day. The findings are as follows:
1.
Causality Link was able to capture news content. On the day when information relating to a company is released, the long portfolio achieved an average daily return of +0.7%, while a return of -0.5% was realised by the short portfolio. This resulted in an overall excess return of +1.3%5. Significant portfolio return was also documented on the following day (0.04%) (see Figure 2). Using Amundi’s signals, one may also earn excess returns by executing a long-short trading strategy on the data’s sub-sets (e.g. fresh/stale news, financial/ESG news), although transaction costs
can be notable.
2.
Market reactions were most significant for news about a company’s future on its publication day (+1.7%) compared to that of its present (+1.2%) and past (+0.4%)6 (see Figure 3). This likely reflects the latter being anticipated before publication day. Also, the reaction to near-term future news is larger than that relating to the long-term future. As the former contains less uncertainty, any changes in near-term forecasted cash flows were discounted less heavily than cash flow changes in the distant future.
3.
Among similar sized companies, news with high media coverage triggered a greater market reaction in comparison to news that received less attention. For instance, a long-short portfolio with high coverage generated an excess return of +2.0% on publication day, a notably larger return than the +0.2% return achieved on a portfolio with low coverage. In addition, fresh news achieved a stronger reaction on publication day, and the following day, than that generated by stale information.
4.
The market reaction was small but positive to both upbeat and negative news regarding ESG issues around the publication date. Also, the market reaction was weaker for company news concerning ESG issues than for information relating to its finances (see Figure 4).
5.
Company size influences the magnitude of the market reaction to news. A long-short strategy focused on large companies generated positive returns on publication day (+0.7%), but this was of a much smaller magnitude compared to the information about mid- and small-cap companies (+1.8%) (see Figure 5). This can be due to limited news flow about the latter, as analysts are less likely to cover them.
We were able to precisely identify the informational content of the news. We showed that stock returns react more strongly to financial news than to ESG news, and to information concerning a company’s future than to that relating to its past.
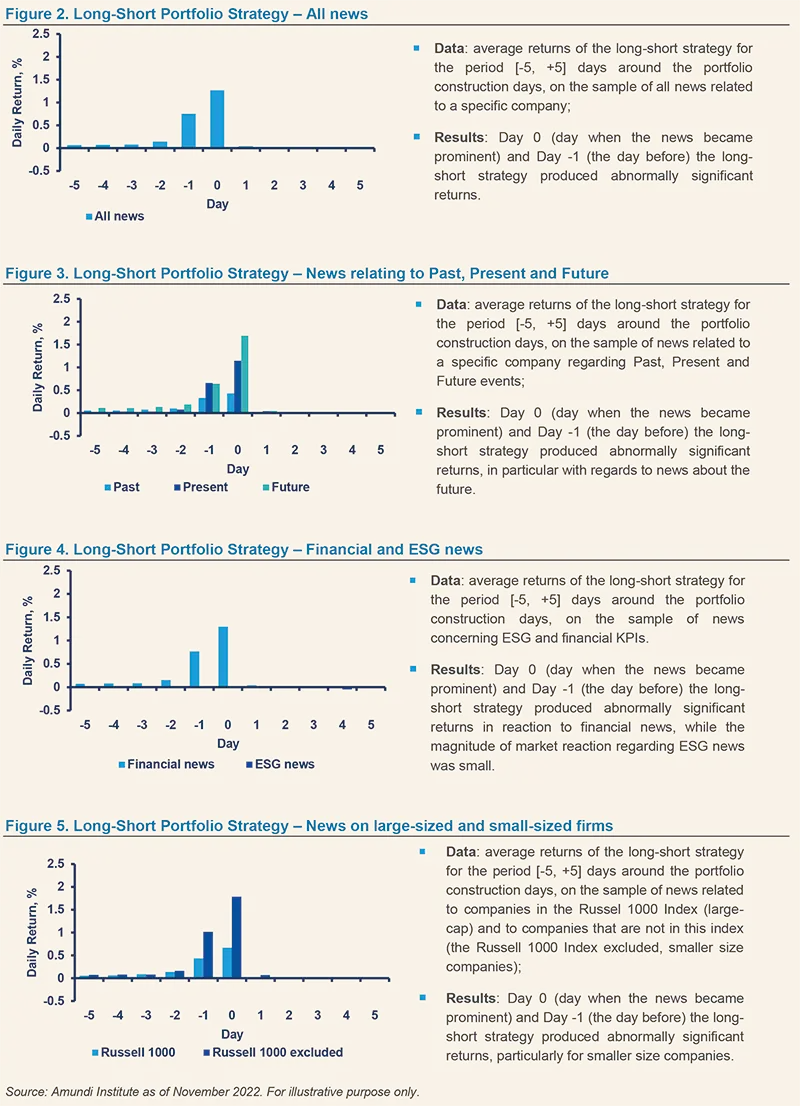
Sources
1. The Causality Link’s AI-powered platform collates millions of documents in real-time to find cause-and-effect statements relating indicators and events. Combining these causal links with predictive analytics provides valuable insights and forecasts for companies and industries that cannot be generated with traditional data.
2. Briere, M., Huynh, K., Laudy, O. and S. Pouget, “What do we learn from a machine understanding news content? Stock market reaction to news”, Amundi Working Paper, forthcoming (2022).
3. Causality Link database contains 1,700 KPIs including financial indicators (e.g., profit, assets, liabilities, market share, etc.) and ESG indicators (e.g., carbon emission, human rights, etc.).
4. Amundi’s methodology is based on an aggregate news signal and data sample that includes all non-microcaps stocks that traded on the AMEX, NYSE and NASDAQ from January 2014 to December 2021.
5. Excess returns are defined according to the Fama and French Three-Factor Model. This builds on the capital asset pricing model (CAPM) by
adjusting for the fact that value and small-cap stocks outperform markets on a regular basis.
6. Return data refers to gross excess returns with no adjustment for transaction costs.
Read more on this subject
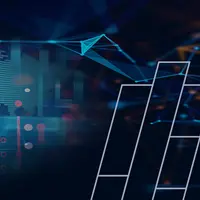