Summary
Executive summary
What is the purpose of this paper?
In the first papers of this series, we addressed the issues of how institutional investors should set their investment objectives – a key element of any Investment Policy Statement (IPS) – and how to articulate their asset allocation across different horizons. Another component of IPS relates to the definition of asset classes included in an investment universe and how to group them. This issue of asset segmentation has meaningful consequences on investment governance and the portfolio construction process. This paper aims to answer the following questions:
- What is the benefit of segmenting your asset universe?
- What are investors’ asset segmentation practices?
- What is the impact of investors’ segmentation decisions on the governance of an allocation process?
- How can asset segmentation be adapted to the risk of rising inflation?
Our answers are based on a combination of financial literature, the findings from investor surveys and from our contact with institutional clients, as well as from our experience in advising them on asset allocation issues.
Who is this paper for?
It is directed towards institutional investors and should be particularly relevant for investment professionals involved in setting strategy or exercising management responsibilities within an investment organisation.
Key messages and recommendations:
- Asset allocation segmentation may be considered sub-optimal by academia, but it is the usual practice among institutional investors. It can be a useful way of viewing a portfolio. It can help the structure of an investor portfolio and its sensitivity to various parameters be understood, as well as helping to enhance its diversification.
- Segmentation is an important decision that investors should address based on their belief of the key return drivers in financial markets.
- The standard approach to asset segmentation is by asset class, while some investors have adopted purpose-driven asset segmentation between safe and growth assets. This can help build a well-diversified asset allocation, in addition to, or in substitution of, traditional asset class segmentation.
- Creating an inflation cluster is sensible when anticipating a scenario where high inflation would be persistent. Asset classes that typically appear in this cluster are inflation-linked bonds and commodities. Mixed assets, such as real estate and infrastructure, also display some sensitivity to high inflation and can be at least partly integrated in this cluster.
- To define such groups, a clustering methodology which distinguishes assets according to their behaviour in different growth and inflation scenarios can be helpful.
This paper and what next
After a short introduction of the benefits and limitations of segmenting a universe of asset classes, this paper will describe different approaches to asset segmentation and outline some governance recommendations. We will then discuss the integration of an inflation asset allocation bucket, designed to cope with the risk of high inflation becoming entrenched. In the final section, we will present an investor case illustrating the quantitative methodology we recommend to define asset clusters. In our upcoming publications, we will focus on issues relating to the management of currencies: to what extent should investors hedge their currency exposure and should such exposure be managed actively or kept stable over time?
“Given we are now firmly in a high-inflation environment, understanding how to reposition asset class segmentation will be key.”
Monica Defend, Head of Amundi Institute
Given we are now firmly in a high-inflation environment, understanding how to reposition asset class segmentation will be key
Benefits and drawbacks of asset segmentation
Despite the drawbacks underlined by academic research, the segmentation of asset classes into broad categories is a common practice within institutions where investment management departments are typically divided and structured by asset class, such as equities, fixed income and alternative investments, and sometimes even by sub-asset classes like regions and sectors. This tends to result in a two-step decision process:
- In the first stage, a central structure or individual, such as the board or the Chief Investment Officer (CIO) typically relying on an investment strategy committee, designs a strategic or medium-term asset allocation at a broad asset class level.
- It then allocates capital to different asset classes, which are delegated to specialised teams or portfolio managers who decide how to invest it. This is justified by the fact that, in order to achieve superior returns through active management, portfolio managers need a high level of specialisation on which they can build an informational advantage.
This two-step process has some drawbacks:
- Firstly, it can lead to diversification losses, as an unconstrained (single-step) solution to portfolio optimisation is likely to deliver results that differ from the optimal linear combination of efficient portfolios in each individual asset class; in this sense, segmenting the asset allocation decision between different sub-decisions is sub-optimal.
- Secondly, there may be differences in risk appetite and investment horizon between the CIO and the specialised teams/asset managers. Portfolio managers’ investment horizons are generally shorter than the CIO’s due to their differing compensation schemes. Moreover, in the case of a defined-benefit pension fund, the liabilities it faces affect the optimal portfolio choice at an aggregate level, but not the choices of unconstrained asset managers, whose return is typically measured against a fixed benchmark.
As such, this two-step process can induce significant costs at an aggregate level[1], leading a number of institutional investors to adopt a Total Portfolio Approach to manage their asset allocation, within which every potential investment is evaluated according to its contribution to the portfolio’s goal. Other solutions exist in order to limit these drawbacks, which we will address in the governance section of this paper, but first let us analyse the different potential approaches to segmentation.
Different approaches to asset segmentation
The main benefit of asset segmentation is to focus the allocation decision process on the key drivers of asset returns. Therefore, investors should spend time on articulating their beliefs about what they consider such drivers to be: broad asset classes, factors, geographies, investment styles or investment purpose (e.g., return, income, hedging).
The traditional segmentation is by asset class, based on the well-established diversification between equities and bonds, with geography often considered as a secondary source of diversification within each major asset class. Some investors also define an ‘alternative assets’ bucket, including private equity, private debt, real estate and infrastructure, even though this means grouping highly diverse types of assets around a common factor: their reduced liquidity compared with traditional assets. This approach to segmentation was challenged during the 2008 crisis, when the expected diversification provided by certain so-called asset classes (hedge funds in particular) proved to be illusory in the context of falling markets.
The following years saw the emergence of factor diversification, which eventually disappointed many investors, not so much due to the failure of this concept, but rather to the dominance of growth strategies that prevailed in a context of easy monetary policy and record-low interest rates, limiting the benefits of this source of diversification.
How do institutions approach the issue? Based on our analysis of the public information provided by large institutional investors and our discussions with a selection of these, we observe that most investors implement some form of asset allocation segmentation. The most common practice seems to be the traditional allocation across asset classes (with a segmentation between equity and fixed income) due to its practical advantages, but a number of institutions have segmented their allocation in terms of economic purpose or risk, encompassing a growth-hedging or a return-income generation segmentation. Pension funds, in particular, usually split their allocation between growth – largely composed of equity-related strategies – and hedging (or safe-haven) assets, composed of high-quality bonds. This approach has a significant overlap with the traditional equity-fixed income allocation split, but it has the advantage of providing investors with more flexibility to allocate to other types of assets that may have equity-like or bond-like features, including real estate or infrastructure for the latter. Some investors, including a major Australian pension fund, have also decided to allocate their portfolio in terms of risk premia which are identified by the potential reward they offer, such as illiquidity, credit and FX carry, rather than by asset class.
In figure 1 we present different examples of purpose-based asset segmentation. In the first one, a major Asian pension fund decided to split its allocation between return enhancement, capital preservation, inflation hedging and day-to-day operation portfolios. In the second one, a major European pension fund split its allocation between a growth and a hedging portfolio.

Some investors have adopted a mixed approach, combining the traditional allocation by asset class with additional categories such as ‘liquidity’, ‘overlay’ or ‘opportunities’. This mixed approach can also combine an allocation to traditional asset classes and its implementation by factors within these. For instance, a given allocation to equities can be implemented by investing in equity factor strategies which, in the case of high-dividend or low-volatility strategies, are designed to help mitigate portfolio volatility.
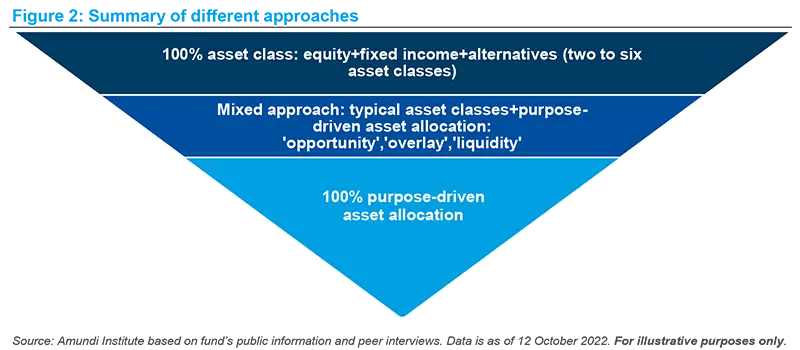
In addition, liquidity can be another segmentation axis. This implies splitting assets between those that are fully liquid – especially during crisis times; those that offer limited liquidity – as is the case of most private assets; and those in-between – such as high-yield debt – whose liquidity depends on market conditions and on the size of trades. Such liquidity segmentation helps ensure that the investor can meet liability requirements while benefiting from the illiquidity premium.
Governance considerations
We will now address the potential consequences of segmentation decisions on investment governance. First and foremost, the type of segmentation chosen by the institution should reflect its beliefs regarding the key drivers of asset class returns, depending on the macroeconomic regime that is deemed likely to prevail. As a result, its definition should be adapted in case of an anticipated change of the macroeconomic regime. Given its importance within the strategic asset allocation (SAA) governance, it should be validated at the highest level of the organisation (board of directors).
Then, we recommend defining a limited number of segments (possibly up to four/five) to avoid complexity linked to excessive granularity and to ensure that selected segments are specific and not highly correlated to each other. These groupings, or clusters, should ideally be statistically independent from each other, or as close to orthogonality as possible. This ensures that asset classes within the same cluster behave similarly and, conversely, that those belonging to different clusters behave differently. Operationally it is better to minimise the number of clusters as the more you create, the more difficult it is to explain and monitor their behaviour over time.
In order to avoid the potential sub-efficiency of asset allocation segmentation compared to a total portfolio approach, a strong central team should be in charge of piloting the portfolio return/risk/liquidity allocation, based on quantitative tools designed to compare asset classes within a coherent framework and to test their behaviour under different scenarios. In this respect, adding an integrated portfolio construction layer to the different allocation buckets can help overcome the limitations of an overly segmented investment approach.
Such a team could also be in charge of organising regular dialogue between specialists of these various asset classes in order to calibrate and structure an inflation cluster, which we believe institutions should set up in anticipation of a structurally higher inflation scenario over the long term, as shown below.
Coping with high and persistent inflation
The definition of segmentation should be revisited as it is likely that we are entering a new economic regime, due to the accumulation of debt and leverage, as well as increasing commodity prices and the pressure to fight inequality. The probability of high and persisting inflation over the long term may call the diversification effect between bonds and equities, which has been the traditional segmentation of asset allocation, into question. As shown in figure 3, the negative bond-equity correlation observed over the recent years has not been systematic in history. There have been periods of positive correlation between equities and bonds, particularly during times of high and volatile inflation.
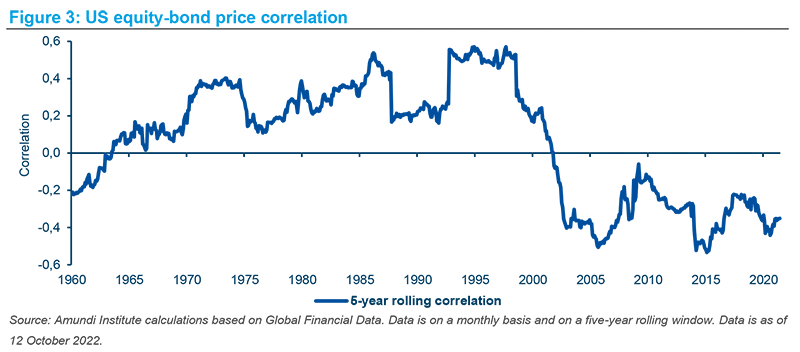
High inflation is negative for bonds, and it also negatively impacts equities, at least in the medium term until indexation mechanisms allow earnings to keep up with the higher nominal growth trend. In this type of environment, it is sensible to diversify allocations beyond the traditional bond-equity segmentation and to define an inflation-hedging category of assets. A number of investors have already implemented this advice by including an inflation-hedging bucket in their allocation, as observed in the previous chapter.
Obvious candidates for this inflation-hedging cluster are inflation-linked bonds and commodities. For some investors, mixed assets such as real estate and infrastructure may also be integrated, as their return is partly impacted by inflation trends. Box 1 provides a short description of our real estate model and illustrates the mixed features of this asset class, which has sensitivities to both growth and inflation and paves the way to a growth-inflation categorisation as outlined in the following chapter.
Box 1: Amundi real estate strategic return model
Our real estate model is based on an interpretation of the cap rates[2] breakdown into nominal economic growth and a risk premium. The former aims to estimate both the level of and the change in rental income, where the long-term interest rate can be approximated by GDP growth plus inflation. The Willkie model[3] demonstrates the impact of the inflation component both on rental yields and on rental income, by using nominal economic growth. In this manner, our model makes the relation with inflation and the macroeconomic environment explicit. This real estate premium includes a component that depends on the equity premium and whose sensitivity is measured by an estimated beta and an illiquidity premium that is applied to unlisted assets. We estimate the average level of leverage for core real estate is 20-30%. This is included in the asset class model as an add-on that can be adapted to an investor’s specifics. It is worth noting that for medium- to long-term horizons, our macro-based relations approach sets a rental yield and incorporates a mean-reversion process to the long-run equilibrium, excluding tactical and valuation considerations. Figure 4 illustrates the breakdown of the real estate return, linked to projections of the following variables: equity (estimated beta in the 0.25-0.50 range), inflation, GDP trend and cycle, liquidity risk model, interest rates and spread (for financing cost), with a focus on the long term. ![]() |
Defining asset clusters: an investor case
Let us continue with some methodological considerations regarding how asset clusters should be set. Our recommended approach is to use clustering methodologies, as they can help define different regimes displaying common patterns, as well as evaluate the degree of segmentation within the universe of asset classes, whose behaviour varies over time, but also depends on the underlying macro regime.
As part of a recent advisory assignment, we assisted an institutional investor in reviewing the classification of assets currently included in its investment universe. To perform this task, we analysed the behaviour of various asset classes in different macroeconomic regimes, defined around the growth and inflation factors we believe are the two major drivers of asset returns. This helped us position asset classes based on their behaviour in different types of scenarios. More details about our methodology can be found in box 2.
Box 2: Amundi methodology
Our methodology combined non-hierarchical clustering algorithms that help define and optimise the split of macroeconomic regimes with hierarchical ones to enable the segmentation of asset classes in a sequential way and obtain appropriate portfolio diversification in each identified regime. In order to identify the relevant macroeconomic factors, we used several of our regime-based tools as starting points, such as:
A hierarchical clustering algorithm was then used to split the investor’s current universe (plus a few additional assets that were being considered for investment) into blocks/clusters and calculated a uniqueness score: the higher the score, the more unique the asset class behaviour. Real and alternative assets were excluded, as daily data is not available for these assets and is a requirement for this kind of analysis. We then defined an indicator (base value) representing the return for an asset class X in a given regime divided by its uniqueness score. Finally, we ranked and rescaled these base values across assets from -1 to 1, which do not imply a preference for an asset class but demonstrate the distribution dispersion of information from opposing regimes. Figure 5 illustrates the categorisation of assets obtained through this analysis. ![]() This figure shows:
|