Summary
- Global investment in Artificial Intelligence (AI) is rapidly increasing across sectors, from manufacturing to services, with a focus on generative AI, leading to higher output in the sectors most involved. Higher capital per worker is also likely to drive future potential growth.
- The debate about AI as a substitute or complement to human labour is misplaced in the short term, as it can be both. The latest wave of AI is different from past technological innovations, as it affects creative and cognitive jobs as well as physical ones and routine cognitive tasks.
- The impact of AI on labour displacement is still uncertain, but reskilling workers will become more important, as some skills become obsolete or need to adapt to work with AI. While there will be some displaced workers, there will also be new jobs and new types of jobs.
- AI has the potential to significantly increase total factor productivity across the economy, impacting a wide range of industries through multiple channels – such as the labour market, investment and productivity – that may not be fully captured by official statistics.
- Social, political and economic hurdles could limit the rapid diffusion and fast adoption of AI across countries. A specific barrier is the huge energy intensity of training generative AI models. AI also raises political concerns about the potential misuse of this technology and the need for regulation to limit its potential manipulation.
Recent advances in the development of Artificial Intelligence (AI) could lead to potentially disruptive changes across a wide range of industries and impact economic activity through multiple channels. AI is widely seen as significantly different from previous technological innovations. But will it significantly increase aggregate economic growth through its impact on labour markets and increases in productivity? And will it enhance the productivity of labour or displace workers? These are important considerations for the sectors that may attract more investment and for economic policy – higher aggregate productivity and higher growth would afford governments more policy space to deal with distributional challenges, such as higher inequality and the displacement of some workers.
How economists think about the aggregate impact of AI
Economists usually model the potential growth of GDP as a function of inputs of capital (K) and labour (L), and the efficiency of the production process (productivity), typically expressed as the Cobb Douglas production function:
𝑌 = 𝐴 𝐿𝑎 𝐾 1−𝑎
Higher inputs generate higher output, as does higher productivity. AI could impact growth not only through higher productivity, but also through its direct impact on labour and capital inputs. Moreover, the impact of AI in the short term may be substantially different from its longer-term impact. The illustration below depicts an approximate distinction between the short-term and long-term effects.
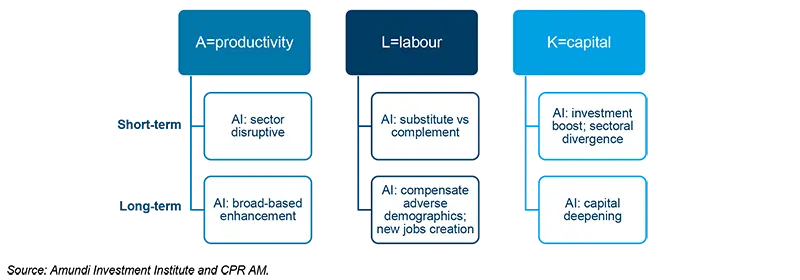
AI and the capital factor: a round of ‘creative destruction’?
Capital investments are indeed a key dimension in the implementation of AI. A recent McKinsey report (The State of AI) shows that in 2022 AI adoption was 2.5 times higher than in 2017. In 2017, 20% of respondents reported the adoption of AI in at least one business area, whereas in 2022 that figure increased to 50%. Consequently, the level of investment in AI has increased alongside its rising adoption. Similarly, in 2017 40% of respondents at organisations using AI reported more than 5% of their digital budgets went to AI, now more than half of respondents reported that level of investment and 63% of respondents said they expected their organisations’ AI investment to increase over the next three years.
Global investment in AI is rising exponentially, including investment in generative AI. Another McKinsey report related to generative AI shows that “funding for generative AI, still only a fraction of the total investment in Artificial Intelligence, is significant and growing rapidly, reaching a total of $12 billion in the first five months of 2023 alone. Venture capital and other private external investments in generative AI increased by an average compound growth rate of over 70% annually from 2017 to 2022. During the same period, investments in Artificial Intelligence overall rose annually by 29%, albeit from a higher base.”
Interestingly, investment in AI is spreading across sectors, moving from manufacturing to services, especially with the latest wave of AI. Back in 2018, according to the McKinsey report, “manufacturing and risk were the two functions in which the largest shares of respondents reported seeing value from AI use”. In 2022, “the biggest reported revenue effects are found in marketing and sales, product and service development, and strategy and corporate finance”. This increase in AI investment is already increasing the output of the sectors most involved, while the higher capital per worker will likely increase future potential growth.
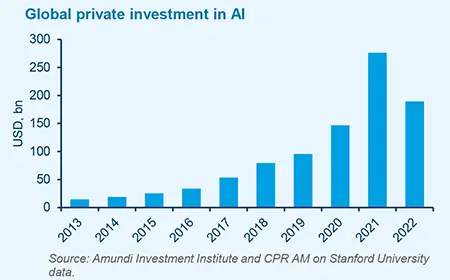
Despite these impressive growth rates, it will take a long time to materially affect the stock of capital in most economies. The evolution of the capital stock depends on two factors: the stock of capital at time t is the sum of the capital stock available at (t-1) minus the depreciation of capital due to deterioration and obsolescence and increments (new investments). If the aggregate capital stock increases this will lead to higher capital per worker and ceteris paribus higher potential growth.
A rapid pace of innovation and evolution in these new AI technologies could make the depreciation rate (proxying the speed at which existing capital may become obsolete) much higher than in the past, thus requiring increasing levels of investments to keep the capital per worker unchanged, implying a higher cost of capital for a given level of available savings in the economy. Thus, the short-term impact could sharply diverge across sectors. This is sometimes referred to as “creative destruction” popularised by the famous economist Joseph Schumpeter.
The impact of AI on the labour market: unclear in the short term, necessary in the long term?
While many think about the most recent release of generative AI, there are various types of AI being used across the value chain, some of which have been in use for a long time:
- ‘Human-in-the-loop’ technologies: software, systems and machines assisting workers (to perform tasks better and more efficiently, with the potential of freeing up time for higher value-added activities);
- ‘No-human-in-the-loop’ technologies: automating processes (e.g., robotics) which remove/substitute labour input.
Therefore, in the short term, the debate about AI as “a substitute versus a complement” to human labour seems misplaced, as it can be both. One peculiar aspect of the most recent wave of AI marks a change from past technological transformations as it expands from “physical automation”, which focuses on physical jobs or routine cognitive tasks, to “cognitive automation”, which sees creative and cognitive jobs being impacted. Instead of the lowest-paid workers being the most affected, now many of the highest-paying occupations could be at risk too.
A recent ECB study1 shows that around 25% of jobs in European countries are in occupations highly exposed to AIenabled automation but “the degree of exposure is as much an opportunity as it is a risk. The outcome for jobs depends on whether the AI-enabled technologies will substitute or complement labour.” It shows that the doommongering about the impact of AI on the labour market is “greatly exaggerated”. Indeed, it demonstrates that in Europe, the subsectors most exposed to deep learning AI (language processing, image recognition, recommendations based on algorithms, fraud detection, etc.) during the 2010s saw their weight in total employment increase. For low- or medium-skilled workers, exposure to AI had no impact on employment. For highly skilled populations, exposure to AI had a significantly positive effect on employment. On average, exposure to AI also had a positive effect on the employment of the younger population. No significant impact has so far been observed on wages. The conclusion of ECB economists is that it is too soon to reach a verdict.
The reskilling of workers will, however, be even more important, as some skills may become obsolete or may need to adapt to operate in conjunction with AI. Historical precedents tell us that technological innovation that initially displaces workers typically raises employment growth over a longer horizon (see paper from Goldman Sachs), and that workers who lose jobs can transition to new jobs and, on average, see an increase in labour income. We think that the present may rhyme with the past: on the one hand, as in every major technological revolution, workers will be displaced, but on the other hand, the technological revolution will also require new jobs and new types of jobs that currently do not exist. However, depending on the scale and the speed of the adoption of AI and the regulatory framework, the short-term impending disruption of the labour market remains highly uncertain.
Moreover, AI – while disruptive in the short-term – may also help address the demographic challenge, especially in advanced economies and some of the larger emerging economies. Against the backdrop of an ageing population, this technology could reduce the need for some types of labour. In the Eurozone, for example, the working-age population is falling by more than a million each year. Japan, China, and South Korea are also facing a similar decline. AI could help fill the gap of “missing workers” and, at the same time, raise labour productivity. Daron Acemoglu and Pascual Restrepo (Review of Economic Studies)2 , found strong empirical evidence that the countries where ageing is the most rapid have invested more in the new technologies of robotisation and automation. They further show that the “ageing” factor alone explains around 35% of the differences in robotics investment between countries, and countries with the most acute ageing dilemma also invest more than the others in other automation technologies.
AI and the impact on productivity: significant but when?
The primary determinant of long-term growth is productivity. For the United States, from WWII until the early 1970s, labour productivity growth averaged over 3% per year. In the early 1970s, productivity growth slowed markedly, posting a rebound in the 1990s but it has been declining dramatically again since the early 2000s.
Productivity growth is generally achieved in three ways: by raising the quality of labour, increasing capital per worker or improving the way labour and capital are combined (total factor productivity). In the 1990s, the surge in productivity growth, which lasted for a decade, was driven primarily by a huge wave of investment in computers and communications, improving both total factor productivity and capital intensity. As highlighted in the box below, the diffusion of new technologies is not necessarily straightforward as there could be hurdles of many types and realising productivity gains at the aggregate economy-wide level could take a long time.
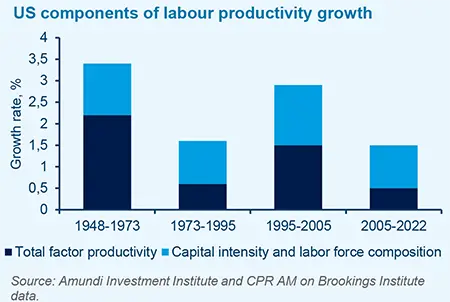
As in the 1990s, AI may significantly increase total factor productivity across the economy, because it has the potential to impact a wide range of industries and do this via multiple channels, such as the labour market, investment and productivity. While part of the economic impact of these new technologies may be measurable (e.g., the new types of jobs created versus the type of jobs lost; R&D expenditure and investment in AI) official statistics may not fully capture the boost to productivity, for example in the service sector and for knowledge workers. We expect the adoption of AI to proceed in three phases:
- “Limited visibility”: a first phase characterised by strong innovation and capital accumulation but with limited visibility on the productivity impact; no widespread adoption, and productivity benefits will be partly offset by losses in some sectors.
- “Broader diffusion”: a second phase, when the cost of using and investing in new technologies falls, the implementation becomes more widespread and increases in productivity spread across the economy. Divergences may remain, but the benefits are more visible.
- “Normalisation”: a third phase, with diminishing marginal returns to further adoption and when the boost to productivity growth tapers off, most likely returning to a longer-term trend.
Estimating the potential impact on productivity is difficult. The emerging literature on estimating the productivity effects of AI is largely sector-specific. Studies that focus on the most recent developments of generative AI – the impact on cognitive workers – find that AI could improve productivity by 10-20%. But these findings are limited to specific jobs or sectors and we think may not extend to the whole economy3. There may, however, be other hurdles – social and political factors, as well as economic barriers – that could limit the rapid diffusion and fast adoption across countries.
How the global context influenced the diffusion of innovations
The speed at which innovations typically spread through society depends not just on their intrinsic benefits, but also on the broader social and economic environment. The low take-up of technological innovations has often been cited as one of the main causes of the weak productivity performance at the beginning of the 21st century (the well-known “productivity paradox”)4 .
Companies’ adoption of new technologies depends, for example, on both human factors (managerial skills and the quality of strategic vision, the level of employee training and suitability of employee skillsets) and market factors (barriers to entry and exit, trade restrictions, the ability to finance innovations, the existence of the infrastructure necessary to use the innovations, tax incentives and bureaucratic hurdles)5 . Similar phenomena affect households’ adoption of new technologies.
Public policies to address such barriers (training programmes, facilitating financing, subsidies, the development of infrastructures, etc.) can help accelerate diffusion, but this often requires an alignment of public and private interests. In some areas – such as the energy transition or strategic autonomy, for example – cooperation between various actors is necessary.
A specific barrier to AI adoption is the huge energy intensity of generative AI models. Some recent research6 shows that training GPT-3, which is a single general-purpose AI program that can generate language and has many different uses, took 1.287 gigawatt hours or about as much electricity as 120 US homes would consume in a year. That training generated 502 tons of carbon emissions, equivalent to that emitted by 110 US cars in a year. Another relative measure comes from Google, where researchers found that Artificial Intelligence made up 10-15% of the company’s total electricity consumption, which was 18.3 terawatt hours in 2021. That would mean that Google’s AI burns around 2.3 terawatt hours annually, about as much electricity each year as all the homes in a city the size of Atlanta.
Further, AI also raises political concerns about potential misuse and the more complex issue of how to regulate its use to limit its potential for manipulation.
Conclusion
In the long term, it is inevitable that AI will be widely adopted, and that it will have a positive impact on productivity and economic growth. Which in turn means that it will be an important consideration for all investors. It could turn out to be a huge gain for countries where the labour force is projected to decline. But investors also need to be mindful that AI will be disruptive in the short term and will adversely affect profitability and returns in a number of sectors. It will also raise challenges for governments on how to deal with displaced labour. Navigating through the short- and medium-term issues that AI raises, to reach the long-term benefits will be a complex, but promising path.
Sources and References
1. ECB, “Reports of AI ending human labour may be greatly exaggerated", ECB research bulletin n°113
2. Acemoglu D. and P. Restrepo, 2022, “Demographics and automation”, Review of Economic Studies, vol. 89(1).
3. Economic theory posits (through Hulten’s theorem) that the impact of a productivity boost in a given sector on aggregate productivity and output is equal to the size of the productivity boost weighted by the relative size of that sector in the economy (in competitive markets). Thus, if we assume AI increases productivity by 10% in one third of the economy over a period of 10 years, aggregate productivity would be 0.3% higher in 10 years. This would represent a very significant increase. Moreover, given the nature of AI, its diffusion across many countries could be much higher than previous technological innovations. It is unlikely to benefit only the innovating countries. This is because AI technologies can, in principle, be rolled out quickly via software and the digital internet infrastructure – its deployment may not require users to invest in expensive infrastructure.
4. Acemoglu, Daron, David Autor, David Dorn, Gordon H. Hanson, and Brendan Price. 2014. "Return of the Solow Paradox? IT, Productivity, and Employment in US Manufacturing." American Economic Review, 104 (5): 394-99. Andrews, D. C. Criscuolo and P. Gal (2016), “The Best versus the Rest: The Global Productivity Slowdown, Divergence across Firms and the Role of Public Policy”, OECD Productivity Working Papers, No. 5.
5. OECD, 2018, “Digital technology diffusion: a matter of capabilities, incentives or both?”, Economics Department working paper n°1476.
6. Patterson D. et al., 2021, “Carbon emissions and large neural network training”
- https://www.ukonward.com/wp-content/uploads/2023/05/Generative-AI-Revolution-Final.pdf
- Brookings Institute, Machines of mind: The case for an AI-powered productivity boom (2003), https://www.brookings.edu/articles/machines-of-mind-the-case-for-an-ai-powered-productivity-boom/
- Capital Economics, AI, Economies and Markets. How artificial intelligence will transform the economy (2023).
- OECD WP No. 288 (2023).
- Mc Kinsey, The economic potential of generative AI, the next productivity frontier (2023).
- Mc Kinsey, The state of AI in 2022—and a half decade in review (2022).
- Goldman Sachs Economic Research (2023), The Potentially Large Effects of Artificial Intelligence on Economic Growth.
- PWC The macroeconomic impact of artificial intelligence (2018).
- https://ourworldindata.org/grapher/corporate-investment-in-artificial-intelligence-by-typ
- https://ourworldindata.org/grapher/private-investment-in-artificial-intelligence-by-focus-area?facet=none
- https://www.ukonward.com/wp-content/uploads/2023/05/Generative-AI-Revolution-Final.pdf
- IMF Blog, TECHNOLOGY’S BIFURCATED BITE (2023) Berg, Papageorgiou, Vaziri.
- IMF Blog, The Macroeconomics of Artificial Intelligence (2023).
- IMF Blog, Rebalancing AI (2023) Acemoglu, Johnson.
- NBER, Generative AI at Work, Brynjolfsson, Li, and Raymond (2023).