Summary
-
Amundi’s research1 highlights that robo-advisors have a positive impact on behaviour and risk-adjusted returns in settings where individuals retain overall control (i.e. automatic portfolio rebalancing is not used)
-
Investors’ attention, trading activities and risk-taking increases, resulting in greater returns (approx. +2% per annum) being achieved. Yet, the negative effect of not implementing automatic rebalancing is very small.
-
The alerts sent by the robots are an effective tool in inducing individuals to rebalance their portfolios towards their target allocations (the probability of rebalancing increases by 29%).
-
Investors are less likely to follow the robot’s recommendations during bear markets. The probability of rebalancing trades being triggered by alerts during a bear market (e.g. October - December 2018) is significantly lower (22.5%) than during other more buoyant market periods (48%).
Our research showed that after using the robo-advisor, individuals were more inclined to invest in equities and follow the robo’s recommendations in rebalancing their portfolios towards their target allocations. These enhanced choices led to higher risk-adjusted returns.
Why Robo-Advisors
Individuals’ decision-making is subject to biases which can result in them focusing on assets they know, avoiding facts that contradict their convictions and engaging in excessive trading activity. To counteract these biases, robo-advisors (often called automated financial advisors) are increasingly gaining traction, and this has triggered questions about investors’ optimal role in the management of their portfolios. A widespread aversion to relying entirely on algorithms has ensured that it is difficult to determine what this level should be and this has led to two types of robo-advisors to coexist in the market place.
Types of Robo-Advisors
The two types of robo-advisors (see figure 1) include the fully automated and automated advisory models.2 The fully automated model avoids the risk of individuals implementing their own biases and/or making choices due to feeling panic in volatile markets. Yet, the academic evidence to support the superiority of fully automated decision, as opposed to those involving humans, is mixed. In specific areas (e.g. legal evaluations), studies have shown that algorithmic conclusions are superior to those made by human. However, others have demonstrated that "mixed" decisions, where humans and machines collaborate, have added more value.3

One of the challenges of robo-advice services is to establish trust. However, research shows that it can be built by allowing humans and robots to interact. For example, enabling users to control the algorithms builds trust, even if they are severely restricted in making modifications. Another way of reassuring investors is to supplement robotic advice with human advice. For example, the human-advisor can intervene in turbulent markets to help individuals to make the right decisions. Finally, offering individuals an explanation of recommendations can also increase trust, even if they are based on a complex model.
Combining digital and human advice provides a world of opportunity – both for wealth managers and investors. Combining the best of both means that those offering advice have the tools to deliver a greater personalised service to more people – a win-win in helping people invest better.
Robo-Advisors Add Value
Amundi research established that the extent to which investors keep an active role in their investment decisions is important in assessing whether and how robo-advisors can improve choices. The research project addressed two main questions.
Q1 | Q2 | |
What significant changes in decisions may occur when a robot provides investment and rebalancing recommendations to investors who still retain full control of their portfolios? |
|
The growing trend in substituting individuals’ decision-making with automatic rebalancing is ensuring that it is important to assess whether significant changes in decisions take place when a robo-advisor is used. Furthermore, our analysis of the effects on behaviour associated with using robo-advisors highlighted the human-robot interactions that can occur over time (e.g. included periods of market shocks or emerging investment opportunities). By assessing these interactions, we were able to compare the behavioural changes associated with the robo-service to counterfactual outcomes investors would have experienced had they fully delegated their decisions.
Research Outline
In partnership with the Toulouse School of Economics, we compared the investment behaviour of a sample of approximately 20,000 robos to 60,000 non-advised clients. The sample was based on the Employee Saving Plans of a large French asset manager who introduced a robo-advisor service in August 2017. This sample was representative of the French population of private sector employees, including small investors who tend to be underrepresented in studies focused on market participants.4
We accessed account level data covering the period from January 2016 to June 2021, aggregated at the monthly level. Our sample included those who:
- Accepted the robo-service (20,000)
- Did not receive an offer to join (20,000 individuals)
- Declined the offer without initiating the profiling process (non-takers - 20,000)
- Initiated the profiling process without subscribing to the service (robo-curious - 20,000).
A particularly interesting feature of our data was that it allowed the comparison of behavioural changes associated with the robot’s take-up to those displayed by the robo-curious. This enabled us to examine characteristics that may induce individuals to be interested in the robo-service, and compare the effects on them of taking-up the robot services relative to those who just observed the robot’s profiling and recommendations.
The robo-advisors questioned individuals on their characteristics, objectives and preferences (investment horizons, age, risk appetites, financial knowledge, experiences etc.), and then offered them a portfolio recommendation that matched their needs. If the portfolio allocation moved too far from the targeted allocation, it delivered email alerts suggesting that the savers rebalance their portfolios to move them back closer to the target allocations. An important feature of the service was that the robot gave advice to investors while leaving them free to follow or ignore the advice.
The robot’s allocation was built among the funds proposed by the employer; that is, access was provided to exactly the same menu of funds with and without the robot. In the absence of the robot, the employees self-managed their portfolios without any dedicated advice. Detailed information on the investment choices was obtained. Specifically, we observed the initial allocations chosen by the employees, new investments, the asset allocation rebalancing activities and withdrawals. The portfolios’ returns and measures of risk were constructed, while information about investors’ activities on the platform were extracted (e.g. trading, number of connections, duration, pages visited). For those who used the robot, we observed the score individuals were given by the robot, their associated profiles and suggested allocations as well as the alerts the robot was sending over time to propose new allocations.
Findings
Our research showed that the robot can significantly affect investors’ decisions while letting them retain control over their portfolios. For example, those who took-up the robot did not view it as a substitute for their own participation. Instead, their attention to their portfolios increased (the number of connections on the platform increased by 0.3 per month, compared to an average of 0.8) and they engaged in greater trading activities after the take-up, triggered by their interactions with the robot.5 Importantly, individuals’ increase in attention persisted even beyond the time of the robo-subscription.
Our analysis also showed the effectiveness of the alerts as a tool for inducing investors to rebalance their portfolios and stay closer to their target allocations. We constructed counterfactual alerts that the robo-curious would have received had they subscribed to the robot, and then showed by comparing the robo-takers to the robo-curious that receiving the alerts increased attention and the propensity to rebalance.
We observed that robo-takers who actually received the alert were 29% more likely to rebalance their portfolios as compared to a baseline probability of rebalancing of 10% for the robo-curious. Specifically, it reduced the distance between current and target equity exposure by 4% relative to the average distance of 11%.
Finally, in our setting, individuals’ demographic characteristics did not appear to be a key determinant of differences in behaviour. The older, richer and male investors were more likely to rebalance on alerts as well as those who had held lower exposures to equity markets in the past. Overall, the magnitudes of these effects were small compared to the average probability of rebalancing upon alerts (about 44%).
Market conditions, however, appeared to be a more important factor in whether the robots’ recommendations were followed. In particular, we showed that they were significantly less likely to be followed during bear market periods (i.e. October - December 2018, February - March 2020). The buying decision, even when guided by a robo-advisor, was undoubtedly more difficult in these circumstances. In fact, we observed that for the average investor the probability of rebalancing on alerts during a bear market was 22.5% compared to 48% in other periods.
These behavioural changes had significant consequences for risk exposures and portfolio returns.6 Our research established that after subscribing to the robot, individuals tended to increase their risk by investing a greater amount in equities (+2.9% compared to an average share of 22%). Importantly, we also observed that relative to the robocurious, robo-takers tended to increase their exposure to risk when subsequent risky returns tended to be larger.
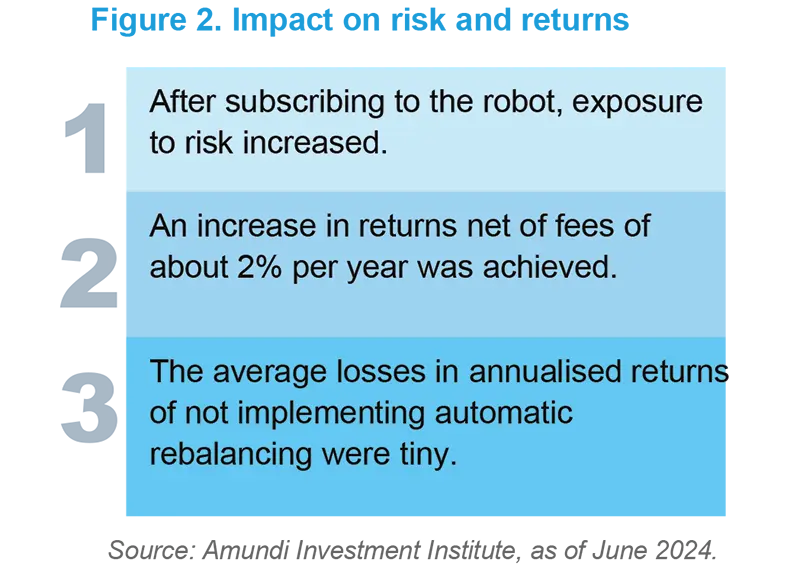
Turning to risk-adjusted returns, they increased by about 2% per year (net of fees) over the studied sample period (2016-2021) after the robo-advisor service was taken-up. This figure was broken down between the static effect brought about by portfolio allocation changes which occurred upon subscribing to the robot, and the dynamic effect of the rebalancing decisions over time. Approximately half of the increase in returns associated with the robo-service could be explained by rebalancing activities. As regards investors’ role in decision-making, we estimated that the potential losses in returns when they retained ultimate control of their portfolios, as opposed to automatic rebalancing taking place, were small (an average loss in annualised returns of about 0.025%).
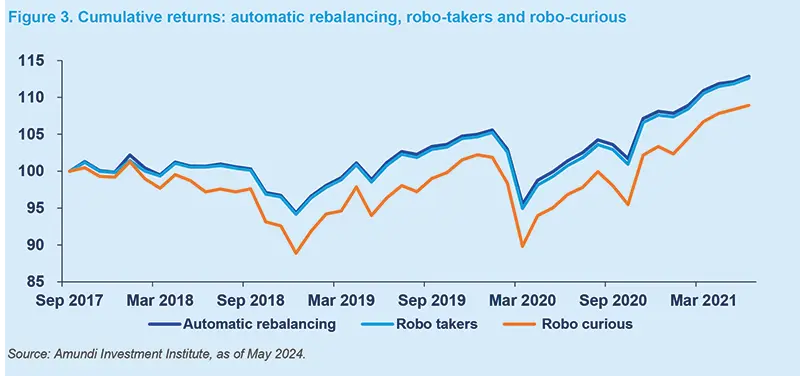
The figure above shows the cumulative returns experienced by various groups over time. The value of the portfolio at the introduction of the robot (September 2017) is normalised to 100 for all groups of investors. On the horizontal axis, time is expressed in months; on the vertical axis, cumulative returns are computed as the average returns experienced by a given group over time (%). The cumulative returns of the automatic rebalancing tend to exceed those of the robo-takers while the robo-takers’ returns also exceed those of the robo-curious. In turn, the magnitude of the differences in returns between the automatic rebalancing and robo-takers is notably smaller than the differences between those of robo-takers and robo-curious. By the end of the sample, the average cumulative returns induced by automatic rebalancing are about 12.85% while those for the robo-takers and robo-curious are approximately 12.6% and 7.03% respectively.
A Tool to Promote Financial Education
Our findings highlight the role of human–robot interactions (i.e. alerts) in the field of personal finance. Investors’ increased attention when using a robo-advisor is important in enhancing returns, a finding that is supported by other studies showing that the opposite is true (low attention can have detrimental effects on returns).7 Furthermore, individuals can learn from the advice offered by the robot as portfolio inertia has tended to be more pronounced for those with limited access to advice, lower wealth and poorer financial literacy.8 This suggests that the robo-service could become a tool to promote financial education which we believe is a key aspect when assessing the long-run consequences of robo-advising.
Today, savers have to make increasingly complex financial decisions, but many of them are not well prepared to do so. At Amundi, we introduced a robo-advisor for our employee savings plans in 2017. Access to automated advice can be a key instrument in promoting financial inclusion.
Other research by Amundi on rob-advisors
- Bianchi M. and Brière M. (2022), “Augmenting Investment Decisions with Robo-Advice”, Working Paper 143 March 2022. Augmenting Investment Decisions with Robo-Advice, Amundi Research Center.
- Brière M. (2021), “Robo-Advising for Small Investors: Evidence from Employee Savings Plans”, Amundi Working Paper 112-2021.
- Bianchi M. and Brière M. (2022), “Robo-advising: Less AI and more XAI? in ‘Machine Learning in Financial Markets: A Guide to Contemporary Practice”, Cambridge University Press.
- Brière M. (2023), Retail Investors’ Behaviour in the Digital Age: How Digitalisation is Impacting Investment Decisions.
Sources
1. Bianchi M. and Brière M. (June 2024), "Human-robot Interactions in Investment Decisions", Amundi Working Paper 150.
2. Bianchi M. and Brière M. (2022), "Robo-Advising: Less AI and More XAI?", in "Machine Learning and Data Science for Financial Markets: A Guide To Contemporary Practices", Ed. Capponi A. and Lehalle C.A., Cambridge University Press. Brière M. (2023), Retail Investors’ Behaviour in the Digital Age: How Digitalisation is Impacting Investment Decisions.
3. Dietvorst B. J., Simmons J. P. and Massey C. (2018), ‘Overcoming algorithm aversion: People will use imperfect algorithms if they can (even slightly) modify them’, Management Science 64(3), 1155–1170. Burton J. W., Stein M.-K. and Jensen T. B. (2020), ‘A systematic review of algorithm aversion in augmented decision making’, Journal of Behavioral Decision Making 33(2), 220–239.
4. The average value of the assets invested in the plan is 37,764 Euros.
5. The focus of the trading activities were the rebalancing trades.
6. In our analysis, we used returns estimated directly from the liquidation value of the various funds.
7. Gargano A. and Rossi A.G. (2018), “Does it pay attention?”, Review of Financial Studies 31 (12), 459-4649.
8. Calvet L.E, Campbell J.Y. and Sodini P. (2009), “Fight and flight? Portfolio rebalancing by individual investors’, Quarterly Journal of economics 124(1), 301-348. Bianchi M. (2018), “Financial literacy and portfolio dynamics”, Journal of Finance 73(2), 831–859.