Summary
- Artificial Intelligence (AI) helps to circumvent some challenges with traditional ESG data.
- Textual and satellite data analysis can discover key ESG risks and opportunities.
- AI contributes to ESG integration by providing an alternative source of data for monitoring ESG reporting.
The Data challenge
ESG data provided by rating agencies is essential, but there are concerns surrounding the quality of this data.
- There is a high degree of subjectivity in the choices made by the rating agencies on ESG criteria. They rely heavily on information provided by the companies being rated.
- Companies’ ESG ratings are reviewed infrequently while the direction of revisions tends to be strongly correlated with financial performance.1
- Large discrepancies among the agencies’ ratings can occur, partly due to the different methodologies used to deal with missing data (see figure 1). These can be large, but, interestingly, research has established that greater ESG disclosure actually leads to greater ESG rating disagreement.2
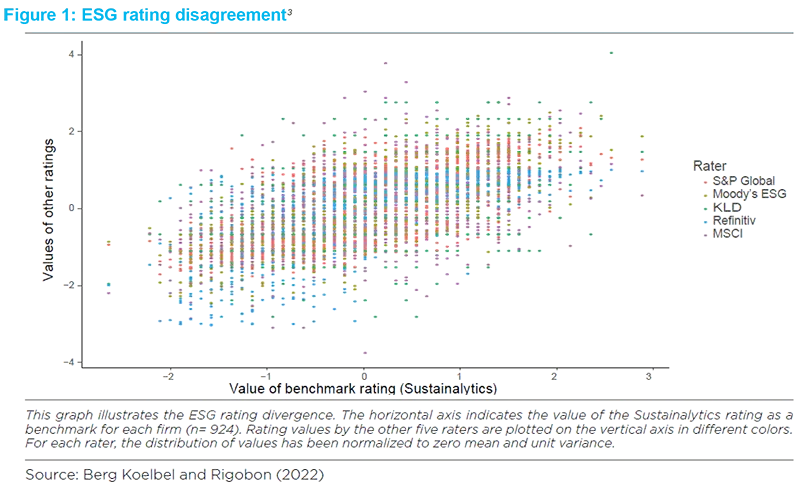
The potential of AI
The good news is that AI tools are available now which can collect and analyse more information on ESG risks and opportunities than ever before. These tools improve the quality of data, analyse it effectively and create new exciting opportunities.
The benefits of AI in ESG investing include:
1 | Provides textual analysis to measure companies’ ESG incidents and commitments
Textual analysis can identify companies’ controversies and important ESG news. ESG data providers (e.g. RepRisk and Truvalue Labs) can use Natural Language Processing (NLP) tools to analyse real-time company information to measure controversies surrounding environmental policies, working conditions, child labour, corruption, etc. For example, RepRisk analyses more than 80,000 media, stakeholders and third party sources daily, detecting incidents that occur in companies’ ESG policies. This type of analysis can be very informative, adding value to ESG investment processes.
Box 1: The Amundi Institute adds value through research
- A recent study4 established that employees are sensitive to news on their companies’ social issues, such as working conditions, when investing in their companies’ shares.
- Using a proprietary algorithm to search newspaper articles, call transcripts and brokers’ notes, the Amundi Institute (in partnership with Causality Link and Toulouse School of Economics) can extract from news statements a company’s name, its Key Performance Indicators (KPIs), the direction of change of those KPIs and the tense of the statement.
- Amundi’s research team investigated how and when new fundamental information made the stock market react. Not only did share prices react to announcements on the day, but they also reacted more strongly to those concerning a company’s future than to those relating to its past achievements. These findings highlighted the strong informational content of the news understood by the software used.5
- NLP techniques are powerful tools which can be used to identify “market narratives” (economic reasoning, geopolitical risks, environmental and social risks, etc.), in our view. A study earlier this year, analysed the informational content of the Global Database of Events, Language and Tone (GDELT). This analysis showed that the information had forecasting power on the U.S. equity market.6
Textual analysis can also shine a lens on companies’ ESG commitments. Research has been conducted focused on climate disclosures.
Box 2: Shinning a lens on ESG commitments
- A AI review examined companies’ compliance with the Task Force Climate-Related Disclosures (TCFD) recommendations.7
- Focused on 10-K reports, research found that transition risk disclosure is increasing faster than physical risk disclosure.8
- Using machine learning to examine 300 European companies, it was determined that disclosure levels are rising at different rates across industries and countries. This suggests that regulatory environments have an important role to play in improving disclosure levels.9
- On examining 800 TCFD-supporting companies, it was evident that some businesses engage in cherry picking as regards climate disclosures, selecting the least relevant information to disclose.10
2 | Collects satellite and sensor data to determine environmental impact and physical risk exposures
Recent years have seen a remarkable increase in satellite and sensor data.11 Possessing a wide geographical coverage, this data can be used to verify companies’ carbon emissions, or to analyse their impact on ecosystems: air pollution, waste production, deforestation, floods etc. This data type can also be a key ingredient of climate risk stress testing models, the findings of which have been very informative, in our view.
Box 3: AI is a key ingredient of climate risk stress testing models
- Gas burning in oil wells to reduce companies’ cost of connecting wells to pipelines was measured.12
- Air pollution caused by forest fires in Indonesia was examined13 while satellite imagery was used to provide estimations for various types of pollutants.14 Satellite data is particularly useful in developing countries, as ground-based air pollution monitoring stations can suffer from government manipulation.
- Satellite data has also been used to monitor deforestation15 and reforestation programs.16
- The impact of floods have been analysed using maps and night-lights data to measure local economic activity.17
3 | Bridges the gaps in company data
AI can help to bridge the gaps in corporate disclosures. While it is considered mandatory for large companies to report on Scope 1 and 2 greenhouse gas (GHG) emissions, reporting on Scope 3 emissions (indirect emissions that occur in a company’s value chain) is optional.18 However, Scope 3 emissions can often be the largest component of companies’ total GHG emissions.
To estimate these, a link for every stage of a company’s industrial processes with its carbon emissions is required, information which is rarely publicly available. To date, data vendors (e.g. CarbonMetrics, Refinitiv ESG Carbon Data) have relied on simple regression models to predict the likely GHG emissions of some companies. A recent study19 used statistical learning techniques to develop models to predict such emissions based on publicly available data. This approach generated more accurate results than previous models.
AI enhances ESG Data but is not without its drawbacks
Ratings based on NLP signals can become public “sentiment” indicators, particularly when the primary source of data comes from social media. For example, a study of the criteria used by Truvalue Labs to assess companies’ ESG risks, demonstrated that it overweighed certain key issues (the ones that generate the most ESG controversies) and that weights change through time.20
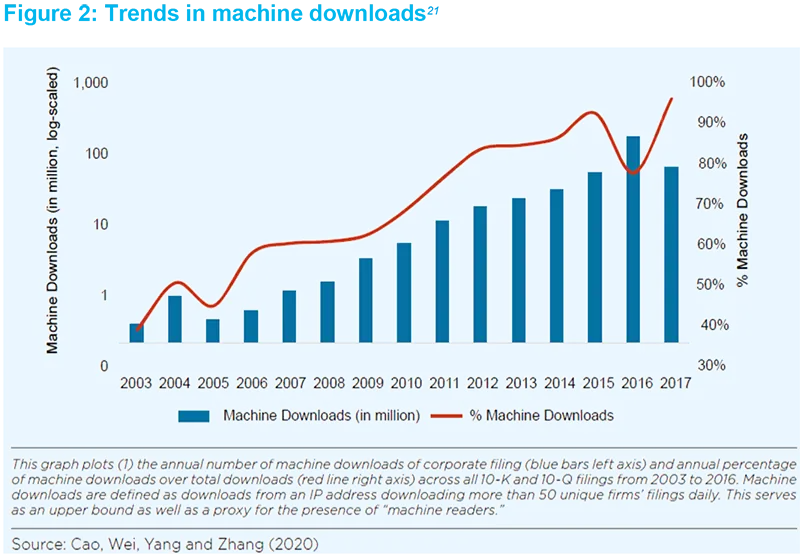
Company disclosures can also be subject to manipulation as more communication is being reshaped in light of AI algorithms.
Managers can learn to avoid words that could be perceived as negative while favouring language preferred by ESG algorithms.
Another issue is a lack of historical data in some instances, which might lead to biases and representatively issues.
Research comparing six physical risk scores showed a low correlation between rating providers, even among those based on similar methodologies.22 In particular, they identified a low correlation between physical risk metrics derived from model-based approaches (i.e. Trucost, Carbon4 Finance and South Pole) and language-based approaches (Truvalue Labs, academic scores).
The future of AI in ESG Investing
AI has the potential to contribute notably to improving the monitoring of ESG reporting and goals. However, there are still challenges in analysing the extensive data available while the choice of one measure over another could have a large impact on the outcome. In the end, a comprehensive investment process should avoid placing too much confidence in a single measure. Furthermore, one also needs to consider the costs of maintaining alternative datasets: not only the costs of acquiring data, but also the investment required to store and integrate these large datasets, activities that might necessitate a dedicated team. Overall, the common consensus is that ESG integration into investment approaches will become more profound and the ability to use robust data will play a major role in that process. Not only can AI help to extract relevant information from existing data sources, it also offers exciting opportunities to create new ones.
Sources and References
1 Berg, F., Fabisik, K., and Z. Sautner, Rewriting history II: The (un) predictable past of ESG ratings, European Corporate Governance Institute–Finance Working Paper (2020).
2 Christensen, D., Serafeim, G., and A. Sikochi, Why is corporate virtue in the eye of the beholder? The case of ESG ratings, Harvard Business School Working Paper (2019).
3 Berg, F., Koelbel, J.F., and R. Rigobon, Aggregate confusion: The divergence of ESG ratings, Review of Finance, forthcoming (2022).
4 Bonelli, M., Brière M., and F. Derrien, “Altruism or Self-Interest? ESG and Participation in Employee Share Plans”, Amundi Working Paper (2022).
5 Briere, M., Huynh, K., Laudy, O., and S. Pouget, What do we learn from a machine understanding news content? Stock market reaction to news, Amundi Working Paper, forthcoming (2022).
6 Blanqué, P., M. Ben Slimane, A. Cherief, T. Le Guenedal, T. Sekine, and L. Stagnol, “Monitoring Narratives: An Application to the Equity Market”, Amundi Working Paper (2022).
7 The Task Force on Climate Related Financial Disclosures (TFCFD) aims to improve and increase reporting of climate-related financial information.
8 Kolbel, J. F., Leippold, M., Rillaerts, J., and Q. Wang., Does the CDS market reflect regulatory climate risk disclosures? SSRN Working Paper (2020).
9 Friederich, D., Kaack, L.H., Luccioni, A., and B. Steffen, Automated identification of climate risk disclosures in annual corporate reports, arXiv preprint arXiv:2108.01415 (2021).
10 Bingler, J. A., Kraus, M., Leopold, M., and N. Webersinke, Cheap talk and cherry picking: What ClimateBERT has to say on corporate climate risk disclosures, Finance Research Letters 47 (2022).
11 Burke, M., Driscoll, A., Lobell, D.B., and S. Errmon, Using satellite imagery to understand and promote sustainable development Science 371(6535) (2021).
12 Bellon, A., Does private equity ownership make firms cleaner? The role of environmental liability risks, ECGI Working Paper (2020).
13 Jayachandran, S., Air Quality and Early-Life Mortality: Evidence from Indonesia’s Wildfires Journal of Human Resources 44(4) (2009).
14 Streets, D. G., Canty, T., Carmichael, G. R., De Foy, B., Dickerson, R. R., Duncan, B. N., Edwards, D. P., Haynes, J. A., Henze, D. K., Houyoux, M. R., Jacob, D. J., Krotkov, N. A., Lamsal, L. N., Liu, Y., Lu, Z.,Martin, R. V., P Ster, G. G., Pinder, R.W., Salawitch, R. J., and K. J., Wecht, Emissions estimation from satellite retrievals: A review of current capability, Atmospheric Environment, 77, 1011–1042 (2013).
15 Tucker, C.J., and J.R.G. Townshend, Strategies for monitoring tropical deforestation using satellite data, International Journal of Remote Sensing 21.6-7 (2000).
16 Li, B., Wang, Y., Wang, W., Wang, C., and A. Lin., Satellite remote sensing analysis to monitor revegetation in the Yangtze River Basin, China. Land Degradation & Development, 33(1), (2022).
17 Kocornik-Mina, A., McDermott, T. K., Michaels, G., and F. Rauch, “Flooded cities”, American Economic Journal: Applied Economics, 12(2) (2020).
18 According to the GHG Protocol of the World Business Council for Sustainable Development (WBCSD), reporting on Scopes 1 and 2 is mandatory, while reporting on Scope 3 is optional.
19 Nguyen, Q., Diaz-Rainez, I., and D. Kuruppuarachchi, Predicting Corporate Carbon Footprints for Climate Finance Risk Analyses: A Machine Learning Approach, Energy Economics 95(3) (2021).
20 Hughes, A., Urban, M.A., and D. Wójcik, Alternative ESG Ratings: How Technological Innovation Is Reshaping Sustainable Investment Sustainability 13.6 (2021).
21 Cao, S., Wei, J., Yang, B., and A.B. Zhang, How to talk when a machine is listening, SSRN Working Paper (2020).
22 Hain, Linda I., Kölbel, Julian F., and M. Leippold, Let’s get physical: Comparing metrics of physical climate risk, Finance Research Letters 46 (2022).